The world of data is filled with promising quotes, such as “information is the oil of the 21st century” or “data is becoming the new raw material of business.” However, the value ingrained in data only becomes vivid when analytics solutions come into play.
Before analytics can start, data needs to be prepared. This stage is a foundation for efficient and effective analysis. And this is where data infrastructure enters the battlefield.
Data architecture is the high-level blueprint that defines how data is collected, stored, integrated, and utilized across an organization. It sets the standards, rules, and policies for managing data assets, ensuring consistency, security, and scalability. Data infrastructure, on the other hand, is the underlying framework of hardware, software, and networks that bring this architecture to life. Together, they form the backbone of modern data-driven enterprises.
In this first article of our Data Architecture Series, we explore how a well-designed data architecture enables an efficient data infrastructure, paving the way for reliable and insightful analytics.
Break down two significant barriers to your data analysis with a robust data infrastructure
Data in an ideal world is perfect and structured, but in reality, several challenges hinder its usability. Two major barriers to an effective data analytics process are:
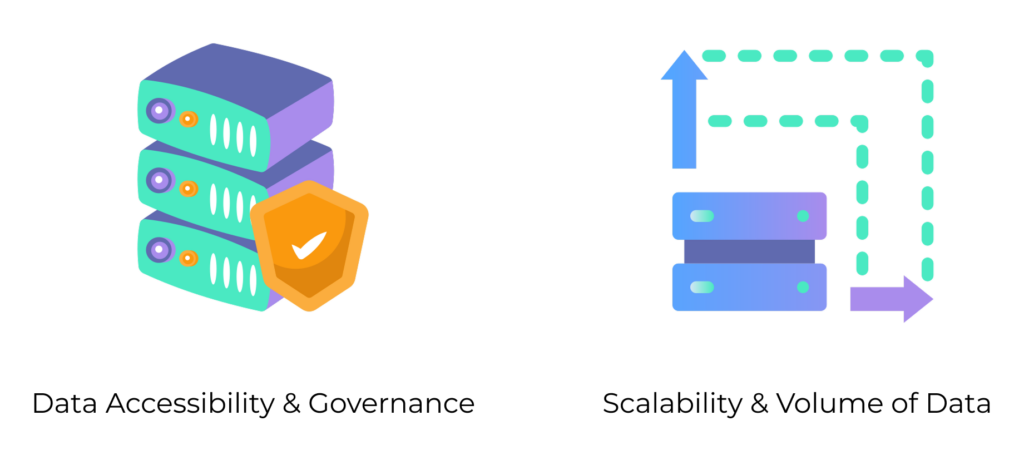
While scalability primarily affects growing companies, accessibility and governance issues impact businesses of all sizes, making them essential considerations in any data architecture strategy.
Data accessibility
No matter how clean and organized your information is, if access is restricted to engineers or data scientists, your organization is not truly data-driven. A recent survey by IDC revealed that 68% of enterprise data goes unused for analytics due to poor accessibility and fragmentation.
Without a well-defined data architecture, companies often struggle with fragmented data silos, leading to inefficiencies in decision-making. Nevertheless it ensures that:
- Data is standardized across the organization.
- Security policies are embedded at every level.
- Access is granted based on roles and responsibilities.
- Metadata management is in place for data lineage and discovery.
For companies aiming for true data democratization, a centralized yet flexible data architecture allows teams to securely access and analyze the information they need, when they need it.
Growing Data Volume
The total amount of data created in 2024 reached 147 zettabytes, while in 2020 it was only 64. This situation became a challenge for data engineers, who are expected to build a new infrastructure to handle such a huge amount of data and get ready for exponential growth in the future in such a short space of time.
The more information you have, the more complex the architecture of the serving infrastructure will be. Data engineers need to seamlessly combine two tasks: rebuild existing data infrastructure and try not to become buried under the ever-increasing data flow.
The amount of data will naturally grow over time — that’s a fact! According to Statista, by 2027, data creation all over the world is expected to increase threefold. Without implementing a proper data infrastructure in your organization, it will be troublesome to grow and stay competitive among other companies. So the best time to start preparing your data infrastructure is now.
Five must-do tips for building a robust data infrastructure
There is no one-size-fits-all solution for data architecture, but focusing on these five areas will help create a robust and scalable foundation:
1. Define your Data Infrastructure Strategy
A concise data infrastructure strategy will save you a lot of effort in the future. First, think of where you will manage your data: in the cloud or on-premises.
The prospect of maintaining your own data center may seem unprofitable, but this is only applicable to small companies. If your organization has enough resources to contain hardware, it may even prove to be more cost-effective. In terms of reliability, there is no difference between the two options.
2. Design a scalable Data Storage System
At the core of data architecture lies data storage design. Organizations must carefully choose between data lakes, data warehouses, or a hybrid model based on their analytical needs:
Data Lake | Data Warehouse | |
Type of data | Structured, semi-structured and raw data from multiple sources | Structured data in quantitative metrics |
Data volumes | Up to hundreds of petabytes (PB) | Mostly in terabytes (TB) |
Data curation | Storing any type of data for big data analytics, machine learning, deep learning, and more | Selected data for read-only queries and data summarization |
Purpose | Big data storage at a very low cost | Data analytics for business intelligence |
Intended users | Data scientists | Business users |
Schema | Schema-on-read | Predefined schemas-on-write |
Cost | Inexpensive | Expensive |
Security | Generally minimal security measures | High grade of data protection |
That said, this doesn’t have to be an either-or decision. There’s also the option to go for a hybrid solution. You can keep the data with minimal business meaning in a lake while storing the useful and relevant data in a warehouse; or use a data lake to collect data and a DWH to structure it. But keep in mind, that these repositories use different technologies: data lake – NoSQL, data warehouse – SQL. So you have to resolve this contradiction when deciding to build your data infrastructure.
3. Ensure Data Quality & Optimization
Data integrity is paramount for accurate analytics. Organizations must establish automated data cleansing and validation mechanisms to maintain high data quality. A robust data architecture should incorporate:
- Real-time data validation pipelines.
- Automated anomaly detection systems.
- Standardized data governance policies for consistency.
Last but not least — be aware of your information quality. The data must always satisfy six conditions:
Completeness. All data sets and data items must be recorded.
Uniqueness. This parameter is kept if data has only been registered once.
Timelessness. This is about how useful or relevant your data is according to its age.
Validity. The data you’ve recorded must reflect the type of data you set out to record.
Accuracy. This metric determines whether the information you hold is correct or not.
Consistency. You can compare data across data sets and media, if it’s all recorded in the same way.
If you find this article interesting, we recommend taking a closer look at
4. Develop a Resilient ETL Pipeline
Extract, Transform, Load (ETL) pipelines serve as the foundation of an organization’s data architecture, enabling seamless data flow between different sources and storage systems. However, businesses often face challenges such as data format inconsistencies, broken data connections, and system integration failures. A well-engineered ETL pipeline ensures that data is ingested, transformed, and stored efficiently, facilitating real-time analytics and decision-making.
5. Implement Strong Data Governance & Security
All the actions above make little sense without proper data governance. It increases efficiency by giving your business a solid database to work from and saving time on correcting the existing data. Besides, it helps to avoid risks associated with dirty and unstructured data and avoid regulatory and compliance issues. A robust data governance framework should address:
- Data ownership and stewardship.
- Access control mechanisms (role-based and attribute-based security).
- Regulatory compliance (GDPR, CCPA, HIPAA).
- Data lineage and cataloging for transparency and traceability.
Solid data infrastructure empowers in-depth analysis
A well-designed data architecture is the foundation of a highly efficient, scalable, and secure data infrastructure, that smooths the road for data science efforts. To benefit from it, you need to care about collecting raw data, cleaning it, and making it accessible.
In the next article of our Data Architecture Series, we will explore the principles of designing scalable and modular data architectures, diving deeper into best practices and emerging technologies.